data lake
What is a data lake?
A data lake is a storage repository that holds a vast amount of raw data in its native format until it is needed for analytics applications. While a traditional data warehouse stores data in hierarchical dimensions and tables, a data lake uses a flat architecture to store data, primarily in files or object storage. That gives users more flexibility on data management, storage and usage.
Data lakes are often associated with Hadoop systems. In deployments based on the distributed processing framework, data is loaded into the Hadoop Distributed File System (HDFS) and resides on the different computer nodes in a Hadoop cluster. Increasingly, though, data lakes are being built on cloud object storage services instead of Hadoop. Some NoSQL databases are also used as data lake platforms.
Why do organizations use data lakes?
Data lakes commonly store sets of big data that can include a combination of structured, unstructured and semistructured data. Such environments aren't a good fit for the relational databases that most data warehouses are built on. Relational systems require a rigid schema for data, which typically limits them to storing structured transaction data. Data lakes support various schemas and don't require any to be defined upfront. That enables them to handle different types of data in separate formats.
As a result, data lakes are a key data architecture component in many organizations. Companies primarily use them as a platform for big data analytics and other data science applications requiring large volumes of data and involving advanced analytics techniques, such as data mining, predictive modeling and machine learning.
This article is part of
What is data architecture? A data management blueprint
A data lake provides a central location for data scientists and analysts to find, prepare and analyze relevant data. Without one, that process is more complicated. It's also harder for organizations to take full advantage of their data assets to help drive more informed business decisions and strategies.
Data lake architecture
Many technologies can be used in data lakes, and organizations can combine them in different ways. That means the architecture of a data lake often varies from organization to organization. For example, one company might deploy Hadoop with the Spark processing engine and HBase, a NoSQL database that runs on top of HDFS. Another might run Spark against data stored in Amazon Simple Storage Service (S3). A third might choose other technologies.
Also, not all data lakes store raw data only. Some data sets may be filtered and processed for analysis when they're ingested. If so, the data lake architecture must enable that and include sufficient storage capacity for prepared data. Many data lakes also include analytics sandboxes, dedicated storage spaces that individual data scientists can use to work with data.
However, three main architectural principles distinguish data lakes from conventional data repositories:
- No data needs to be turned away. Everything collected from source systems can be loaded and retained in a data lake if desired.
- Data can be stored in an untransformed or nearly untransformed state, as it was received from the source system.
- That data is later transformed and fit into a schema as needed based on specific analytics requirements, an approach known as schema-on-read.
Whatever technology is used in a data lake deployment, some other elements should also be included to ensure that the data lake is functional and that the data it contains doesn't go to waste. That includes the following:
- A common folder structure with naming conventions.
- A searchable data catalog to help users find and understand data.
- A data classification taxonomy to identify sensitive data, with information such as data type, content, usage scenarios and groups of possible users.
- Data profiling tools to provide insights for classifying data and identifying data quality issues.
- A standardized data access process to help control and keep track of who is accessing data.
- Data protections, such as data masking, data encryption and automated usage monitoring.
Data awareness among the users of a data lake is also a must, especially if they include business users acting as citizen data scientists. In addition to being trained on how to navigate the data lake, users should understand proper data management and data quality techniques, as well as the organization's data governance and usage policies.
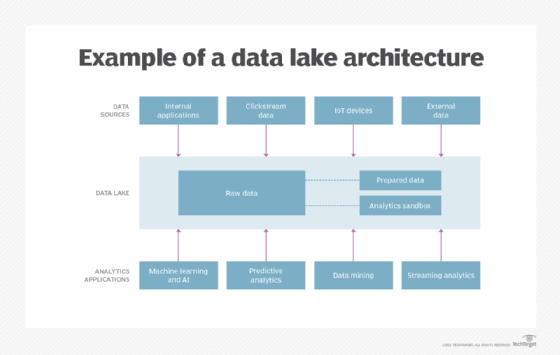
Data lake vs. data warehouse
The biggest distinctions between data lakes and data warehouses are their support for data types and their approach to schema. In a data warehouse that primarily stores structured data, the schema for data sets is predetermined, and there's a plan for processing, transforming and using the data when it's loaded into the warehouse. That's not necessarily the case in a data lake. It can house different types of data and doesn't need to have a defined schema for them or a specific plan for how the data will be used.
To illustrate the differences between the two platforms, think of an actual warehouse versus a lake. A lake is liquid, shifting, amorphous and fed by rivers, streams and other unfiltered water sources. Conversely, a warehouse is a structure with shelves, aisles and designated places to store the items it contains, which are purposefully sourced for specific uses.
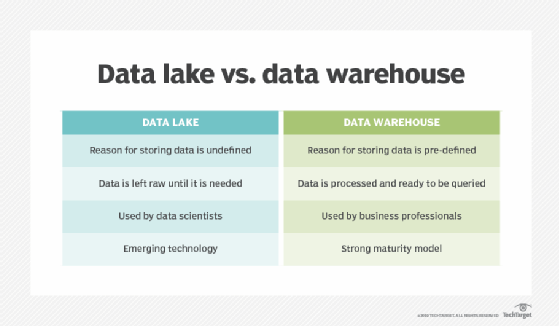
This conceptual difference manifests itself in several ways, including the following:
Technology platforms. A data warehouse architecture usually includes a relational database running on a conventional server, whereas a data lake is typically deployed in a Hadoop cluster or other big data environment.
Data sources. The data stored in a warehouse is primarily extracted from internal transaction processing applications to support basic business intelligence (BI) and reporting queries, which are often run in associated data marts created for specific departments and business units. Data lakes typically store a combination of data from business applications and other internal and external sources, such as websites, IoT devices, social media and mobile apps.
Users. Data warehouses are useful for analyzing curated data from operational systems through queries written by a BI team or business analysts and other self-service BI users. Because the data in a data lake is often uncurated and can originate from various sources, it's generally not a good fit for the average BI user. Instead, data lakes are better suited for use by data scientists who have the skills to sort through the data and extract meaning from it.
Data quality. The data in a data warehouse is generally trusted as a single source of truth because it has been consolidated, preprocessed and cleansed to find and fix errors. The data in a data lake is less reliable because it's often pulled in from different sources as is and left in its raw state without first being checked for accuracy and consistency.
Agility and scalability. Data lakes are highly agile platforms: Because they use commodity hardware, most can be reconfigured and expanded as needed to meet changing data requirements and business needs. Data warehouses are less flexible because of their rigid schema and prepared data sets.
Security. Data warehouses have more mature security protections because they have existed for longer and are usually based on mainstream technologies that likewise have been around for decades. But data lake security methods are improving, and various security frameworks and tools are now available for big data environments.
Because of their differences, many organizations use both a data warehouse and a data lake, often in a hybrid deployment that integrates the two platforms. Frequently, data lakes are an addition to an organization's data architecture and enterprise data management strategy instead of replacing a data warehouse.
Cloud vs. on-premises data lakes
Initially, most data lakes were deployed in on-premises data centers. But they're now a part of cloud data architectures in many organizations.
The shift began with the introduction of cloud-based big data platforms and managed services that incorporate Hadoop and Spark, plus various other technologies. In particular, cloud platform market leaders AWS, Microsoft and Google offer big data technology bundles: Amazon EMR, Azure HDInsight and Google Dataproc, respectively.
The availability of cloud object storage services, such as S3, Azure Blob Storage and Google Cloud Storage, gave organizations lower-cost data storage alternatives to HDFS, which made data lake deployments in the cloud more appealing financially. Cloud vendors also added data lake development, data integration and other data management services to automate deployments. Even Cloudera, a Hadoop pioneer that still obtained about 90% of its revenues from on-premises users as of 2019, now offers a cloud-native platform that supports both object storage and HDFS.
What are the benefits of a data lake?
Data lakes provide a foundation for data science and advanced analytics applications. By doing so, they help enable organizations to manage business operations more effectively and identify business trends and opportunities. For example, a company can use predictive models on customer buying behavior to improve its online advertising and marketing campaigns. Analytics in a data lake can also aid in risk management, fraud detection, equipment maintenance and other business functions.
Like data warehouses, data lakes also help break down data silos by combining data sets from different systems in a single repository. That gives data science teams a complete view of available data and simplifies the process of finding relevant data and preparing it for analytics uses. It can also help reduce IT and data management costs by eliminating duplicate data platforms in an organization.
A data lake also offers other benefits, including the following:
- It enables data scientists and other users to create data models, analytics applications and queries on the fly.
- Data lakes are relatively inexpensive to implement because Hadoop, Spark and many other technologies used to build them are open source and can be installed on low-cost hardware.
- Labor-intensive schema design and data cleansing, transformation and preparation can be deferred until after a clear business need for the data is identified.
- Various analytics methods can be used in data lake environments, including predictive modeling, machine learning, statistical analysis, text mining, real-time analytics and SQL querying.
What challenges do data lakes pose?
Despite the business benefits that data lakes provide, deploying and managing them can be a difficult process. These are some of the challenges that data lakes pose for organizations:
- Data swamps. One of the biggest challenges is preventing a data lake from turning into a data swamp. If it isn't set up and managed properly, the data lake can become a messy dumping ground for data. Users may not find what they need, and data managers may lose track of data that's stored in the data lake, even as more pours in.
- Technology overload. The wide variety of technologies that can be used in data lakes also complicates deployments. First, organizations must find the right combination of technologies to meet their particular data management and analytics needs. Then they need to install them, although the growing use of the cloud has made that step easier.
- Unexpected costs. While the upfront technology costs may not be excessive, that can change if organizations don't carefully manage data lake environments. For example, companies may get surprise bills for cloud-based data lakes if they're used more than expected. The need to scale up data lakes to meet workload demands also increases costs.
- Data governance. One of the purposes of a data lake is to store raw data as-is for various analytics uses. But without effective governance of data lakes, organizations may be hit with data quality, consistency and reliability issues. Those problems can hamper analytics applications and produce flawed results that lead to bad business decisions.
Data lake vendors
The Apache Software Foundation develops Hadoop, Spark and various other open source technologies used in data lakes. The Linux Foundation and other open source groups also oversee some data lake technologies. The open source software can be downloaded and used for free. But software vendors offer commercial versions of many of the technologies and provide technical support to their customers. Some vendors also develop and sell proprietary data lake software.
There are numerous data lake technology vendors, some offering full platforms and others with tools to help users deploy and manage data lakes. Some prominent vendors include:
- AWS. In addition to Amazon EMR and S3, it has supporting tools like AWS Lake Formation for building data lakes and AWS Glue for data integration and preparation.
- Cloudera. Its Cloudera Data Platform can be deployed in the public cloud or hybrid clouds that include on-premises systems, and it's supported by a data lake service.
- Databricks. Founded by Spark's creators, it offers a cloud-based data lakehouse platform that combines elements of both data lakes and data warehouses.
- Dremio. It sells a "SQL lakehouse" platform that supports BI dashboard design and interactive querying on data lakes and is also available as a fully managed cloud service.
- Google. It augments Dataproc and Google Cloud Storage with Google Cloud Data Fusion for data integration and a set of services for moving on-premises data lakes to the cloud.
- HPE. The HPE GreenLake platform supports Hadoop environments in the cloud and on premises, with both file and object storage and a Spark-based data lakehouse service.
- Microsoft. Along with Azure HD Insight and Azure Blob Storage, it offers Azure Data Lake Storage Gen2, a repository that adds a hierarchical namespace to Blob Storage.
- Oracle. Its cloud-based data lake technologies include a big data service for Hadoop and Spark clusters, an object storage service and a set of data management tools.
- Qubole. The cloud-native Qubole data lake platform provides data management, engineering and governance capabilities and supports various analytics applications.
- Snowflake. While it's best known as a cloud data warehouse vendor, the Snowflake platform also supports data lakes and can work with data in cloud object stores.